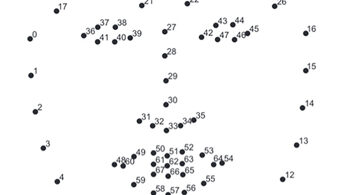
There has been an accelerated growth and application of artificial intelligence over the past decade. Facial recognition software continues to undergo significant advances each year, ranging from personal technology use, such as unlocking a mobile phone or laptop, to government security (i.e., CCTV, passports, biometrics, and border control). Facial recognition uses stored images to identify and match newly encountered images. This process was previously completed in 2-dimensions, which relied on similar framing, illumination, and poses to accurately match new images with an existing database. The advent of 3-dimensional recognition allows analyses to differentiate physical facial features with less reliance on image capture.
Corrective jaw surgery, formally known as orthognathic surgery (OGS), is a procedure that involves repositioning the maxilla and/or mandible to achieve a favourable occlusion. OGS can have significant changes on the vertical, transverse, and anterior-posterior position of the facial skeleton and overlying soft tissues. (Fig. 1) Most orthognathic procedures result in changes to the lower two thirds of the face. There are few studies that have investigated the effects of OGS on the accuracy of facial recognition. Patients undergoing OGS often ask if new identification, such as passports or licences, are required postoperatively.
Fig. 1
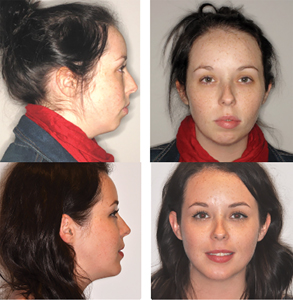
It is hypothesised that OGS alters soft tissue landmarks analysed by facial recognition. These changes may vary depending on the type of surgery (single, double, or triple jaw surgery) as well as the magnitude and direction of surgical movements. Understanding the accuracy of facial recognition following OGS has clinical implications related to patient counselling regarding personal technology use and biometric security. The purpose of this article is to review the current body of evidence in this field, propose a research idea from our centre, and present preliminary results.
Body of Evidence
The current literature has focused on facial recognition in surgical patients following cosmetic procedures, with few studies investigating facial recognition after OGS.1–5 Gupta et al. conducted a systematic review of 39 studies using different facial recognition algorithms (appearance-, feature- and texture-based) to identify patients after various surgical procedures, such as skin resurfacing, malar augmentation, rhinoplasty, blepharoplasty, rhytidectomy, facial reanimation, facial feminization, and OGS.5 The reported recognition accuracy ranged from 19.1% to 85.35%. In the computer science literature, a landmark study conducted by Singh et al. investigated the performance of facial recognition algorithms on 900 patients who underwent cosmetic surgery (rhinoplasty, blepharoplasty, genioplasty, otoplasty, malar implants, rhytidectomy, skin resurfacing and lip augmentation).6 The authors reported poor levels of identification performance and strongly suggested further research in this field. They cautioned the potential misuse of elective surgical changes by individuals attempting to conceal their identity to commit fraud or evade law enforcement.
In a letter to the editor published in the British Journal of Oral and Maxillofacial Surgery, Ryan et al. noted that OGS may invalidate biometric passports and proposed that such changes should be reviewed during the informed consent process.7 Keshtgar et al. surveyed 50 patients following OGS and found that six had difficulty passing through airport security checks (human and automated passport security) due to significant facial changes.8 Four patients underwent Le Fort 1 (LF1) and bilateral sagittal split osteotomy (BSSO) while the remaining two patients received isolated LF1 or BSSO. In the orthodontic literature, Dragon et al. demonstrated that facial recognition scores were significantly lower for patients who underwent double jaw surgery (LF1 and BSSO) compared to patients who received an isolated BSSO.9 Kim et al. analysed 75 patients who underwent OGS and 10 control participants using two facial recognition programs.10 Similarity scores were higher for the Face X program which utilizes a larger number of landmarks during analysis. (Fig 2) For both programs, the similarity scores were highest for the control group and single jaw surgery compared to double jaw surgery for mandibular prognathism and facial asymmetry. Using landmark-based facial recognition, Urbanová et al. demonstrated misclassification of greater than one third of a patient population (n=50) following OGS.11 Changes in features of the midface and lower facial third resulted in misclassification, while landmarks in the upper face facilitated correct identification.
Fig. 2
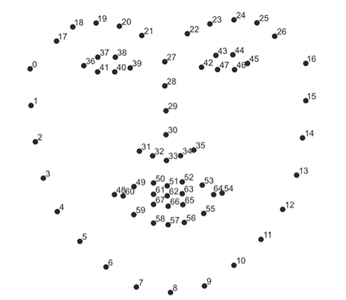
Research Proposal
The research proposal is designed to evaluate if facial recognition software can accurately match pre-surgical images to corresponding post-surgical images (lateral and frontal views) for patients who have undergone various orthognathic surgical procedures. The primary outcome variable is facial recognition accuracy after different orthognathic procedures (LF1, BSSO, genioplasty). It is hypothesized that the magnitude and direction of surgical movement will influence facial recognition. Certain procedures may introduce a higher level of complexity for facial recognition software and a resultant decrease in accuracy during analysis. A secondary aim of the study is to determine if machine learning can improve the accuracy of facial detection and recognition in the context of facial changes associated with OGS.
Preliminary Results
Preoperative images (lateral and frontal views at rest) of 50 cases were evaluated for facial detection and recognition using the Haar Cascade Classifier (HCC) and VGGFace model. The HCC is a machine-learning technique that has been trained for facial detection.12 It applies transfer learning to improve accuracy. The VGGFace2 ResNet50 is used to analyze lateral views. Initial testing has demonstrated reliable facial recognition of frontal views with poor performance on lateral evaluation.13 Following this initial training of the models, pre- and post-surgical images of 500 cases will be uploaded from the senior author’s patient log to further test the reliability and feasibility of the model in facial recognition. Once the models are tested and reliability is verified, a mixture of cases (LF1, BSSO, genioplasty) with different magnitudes and directions of surgical movements will be analyzed to determine the factors influencing accuracy of facial recognition.
Oral Health welcomes this original article.
†Corresponding Author:
Marco Caminiti, Associate Professor, Head and Program Director, Oral and Maxillofacial Surgery, University of Toronto, 124 Edward Street Room 145, M5G 1G6, Toronto, Ontario, Canada. Email: marco.caminiti@utoronto.ca
Disclosing information: None to disclose.
References
- Bouguila, J. & Khochtali, H. Facial plastic surgery and face recognition algorithms: Interaction and challenges. A scoping review and future directions. Journal of Stomatology, Oral and Maxillofacial Surgery vol. 121 696–703 (2020).
- Zuo, K. J., Saun, T. J. & Forrest, C. R. Facial Recognition Technology: A Primer for Plastic Surgeons. Plastic and Reconstructive Surgery vol. 143 1298E-1306E (2019).
- Nappi, M., Ricciardi, S. & Tistarelli, M. Deceiving faces: When plastic surgery challenges face recognition. Image Vis Comput 54, 71–82 (2016).
- Sabharwal, T. & Gupta, R. Deep facial recognition after medical alterations. Multimed Tools Appl 81, 25675–25706 (2022).
- Gupta, N., Hughes, S. J., Chirwa, R. & Cheng, Q. Facial Recognition Software Use on Surgically Altered Faces: A Systematic Review. Journal of Craniofacial Surgery vol. 33 2443–2446 (2022).
- Singh, R. et al. Plastic surgery: A new dimension to face recognition. IEEE Transactions on Information Forensics and Security 5, 441–448 (2010).
- Ryan, P. J., Turner, M. J. A., Gibbons, A. J. & Ricanek, K. Orthognathic surgery and the biometric e-passport: A change in surgical practice. British Journal of Oral and Maxillofacial Surgery vol. 52 384 (2014).
- Keshtgar, S., Keshtgar, A., Mistry, P. & Shakib, K. Assessing facial recognition after orthognathic surgery at automated border controls in airports. British Journal of Oral and Maxillofacial Surgery 57, 536–538 (2019).
- Dragon, C. B. et al. The effect of orthognathic surgery on facial recognition algorithm analysis. American Journal of Orthodontics and Dentofacial Orthopedics 158, 84–91 (2020).
- Kim, W. Y. & Han, S. J. Changes in a facial recognition algorithm following different types of orthognathic surgery: a comparative study. J Korean Assoc Oral Maxillofac Surg 48, 201–206 (2022).
- Urbanová, P., Eliášová, H. & Dostálová, T. How accurate is forensic facial identification of surgically altered faces? Australian Journal of Forensic Sciences (2022).
- OpenCV: Cascade Classifier. https://docs.opencv.org/3.4/db/d28/tutorial_cascade_classifier.html.
- Zhang. R, Hsu, M, Bai, C & Khan, M. Facial recognition for post-dental reconstruction surgery patients April 2023. ECE496, University of Toronto, student project. Supervisor: Prof D. Hatzinakos
About the Authors
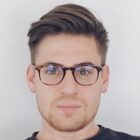
Andrew Lombardi is an Oral and Maxillofacial Surgery PGY-1 resident, University of Toronto.
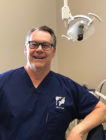
Bruce Pynn is the Chief of Dentistry/ Oral and Maxillofacial Surgery at Thunder Bay Regional Health Sciences Centre and Assistant Professor at the Northern Ontario School of Medicine.
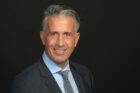
Marco Caminiti is an Associate Professor at the University of Toronto. He is the Head and Program Director of the Oral and Maxillofacial Surgery Residency Program at the University of Toronto and Head of OMFS at Humber River Hospital.