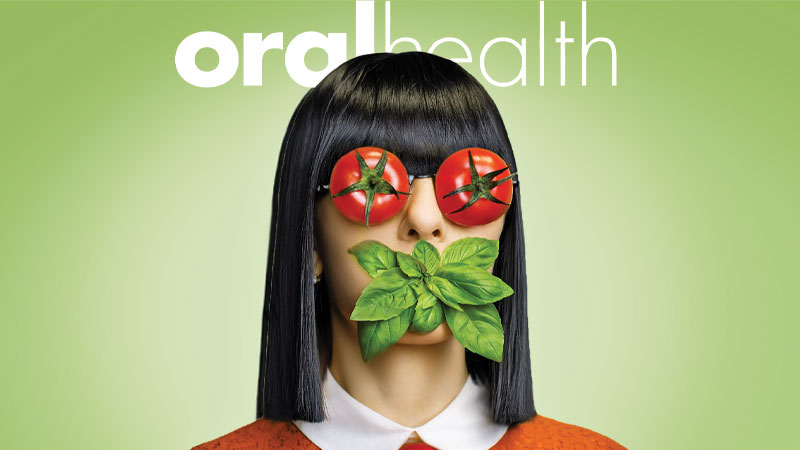
Abstract
Behcet’s disease is a rare autoimmune disorder with nonspecific clinical manifestations that can pose diagnostic challenges for healthcare professionals. This article describes a case in which an Artificial Intelligence (AI) tool (ChatGPT), an advanced language artificial intelligence model, played a pivotal role in aiding a periodontist in diagnosing a patient with Behcet’s disease.
The patient presented with recurring oral ulcers and complained of facial rash and fevers; the patient was refractory to prior treatment. These are common symptoms of Behcet’s disease but can also indicate various other conditions. Seeking to bring the patient some answers, the periodontist turned to AI for assistance and landed upon the differential diagnosis of Behcet’s disease. This case demonstrates the potential of utilizing advanced language models like ChatGPT as a supportive tool in complex medical diagnostics. While such AI models cannot replace the expertise of medical professionals, they can serve as valuable resources for information retrieval, hypothesis generation, and differential diagnosis formulation. As AI technology evolves, healthcare practitioners must integrate these tools responsibly to enhance patient care and outcomes.
Medical learners are often taught, “When you hear hoofbeats, think horses, not zebras,” a doctor first considers what is more common when considering a diagnosis. There is power and validity in using epidemiological data to guide clinical decision-making. However, it can also mean that rare syndromes and diseases may go unrecognized and underdiagnosed. This is an avenue where AI can remind clinicians to keep a broader range of differential diagnoses, which can be narrowed down with clinical impressions and laboratory testing.
In November 2022, OpenAI released ChatGPT, an AI language model. The platform acquired an unprecedented one million users less than a week after launching (Duarte, 2023) and has more than 100 million active users globally. ChatGPT relies on its massive training data to understand the context of a user’s input and generate a relevant and coherent response. The model was trained using ‘zero-shot learning,’ meaning that provided a description, it can even attempt tasks for which it has not explicitly been trained (OpenAI, 2023). Clinical AI and the potential applications of AI as a tool for clinicians have been welcomed with excitement and skepticism by medical professionals.
We present a case study involving a patient who will be referred to as CR, presenting to a periodontist with recurrent mouth ulcers. A biopsy of the affected area yielded pathological findings consistent with an aphthous ulcer. CR had previously sought treatment for these mouth ulcers, fevers, and facial rashes, but prior physicians could not find the underlying issue. After inputting the presenting symptoms of recurrent oral ulcers, a facial rash, and fever into the AI tool, the periodontist was introduced to diagnose Behcet’s disease. On follow-up, the periodontist reviewed the pathology findings and common symptoms of Behcet’s disease with CR. CR had all common clinical symptoms, including mucocutaneous lesions in the mouth and genitals, facial rash, blurriness, arthritis, headache, inflammatory bowel disease, and fever.
Behcet’s disease is an uncommon vasculitis without giant cell formation and necrotization of vessels (Melikoglu et al., 2008). Type 1 Helper T cells (TH1) are considered the key cellular mediator of this disease, resulting in inflammatory cytokines including IL-1, IL-8, IL-12, IL-17, IL-37, and TNF. Increased levels of these cytokines are associated with increased severity (Zouboulis et al., 2003). There are no specific indicators for Behcet’s disease to date (Adil et al., 2023), and it is diagnosed based on clinical presentation. Based on data from the United States, Behcet’s disease has a prevalence of 5.2 out of 100,000 (Calamia et al., 2009); it is not something that clinicians would think of when presented with patients with CR’s clinical picture due to the paucity of cases. This is a perfect example of how AI can guide clinicians’ decision-making to improve patient care. A simple input with CR’s clinical situation into the AI tool provided a differential diagnosis, including the “rare zebra” of Behcet’s disease. Ultimately, the clinician used the AI tool’s response to a patient’s symptoms, laboratory testing, and clinical expertise to rule out more common diseases like herpes simplex infection and efficiently make a more well-informed final diagnosis.
Case Study Description
CR is a 59-year-old female presenting to a periodontal clinic for a biopsy of a recurring mouth ulcer in May 2023 after a referral from her dentist. At the time, she also had a red facial rash and complained of fevers. CR had unsuccessfully sought medical management for these symptoms. She had the buccal surface of her mouth near tooth 37 biopsied without issue. The biopsy report showed she had chronically inflamed granulation tissue consistent with an aphthous ulcer. Before CR’s follow-up appointment to review biopsy results, the periodontist put CR’s symptoms (facial rash and recurring oral ulcers) into the AI tool, identifying the symptoms as indicators of Behcet’s disease (See specific prompt and response in Appendix A). When reviewing the biopsy results with the patients at the follow-up for the biopsy, the periodontist also asked CR whether she had experienced the typical clinical symptoms associated with Behcet’s disease. CR confirmed having recurring oral ulcers (new ulcer on tongue), dyspareunia (indicative of genital ulcers), blurry vision, facial rash, claudication, arthritic pain, fevers, gastrointestinal symptoms, and headaches. Given that Behcet’s disease is diagnosed based on clinical presentation, the periodontist forwarded the presentation and suspected diagnoses to CR’s family physician for further evaluation. The patient was prescribed a steroidal mouthwash to reduce the reoccurrence of mouth ulcers.
Discussion
Advantages of Using AI
It is estimated that medical knowledge doubles every 73 days (Densen, 2011). At this rate, it is impossible for a clinician to keep up to date with the latest advances and findings, especially information about diseases that they do not treat or diagnose regularly. However, generative AI platforms, such as ChatGPT, can double their power and effectiveness every six to ten months (Sevilla, 2022). ChatGPT and other Large Language Models (LLMs) are trained on massive amounts of data. LLMs can identify patterns and structures and use their contextual understanding to draw conclusions and compile their findings as text responses. In our case study, ChatGPT, when provided with the symptoms the patient was experiencing, could engage in natural language processing tasks and provide the clinician with a list of possible diagnoses. Ultimately, this facilitated the clinician to make an informed diagnosis by exposing them to previously unexplored diseases.
Unlike a search engine that retrieves and points users to content, ChatGPT generates responses based on this training data set and the input it receives. All responses generated by ChatGPT are based on the abstract relationship between word tokens in the neural network (OpenAI, 2023). It is not permitted to access external sources of information. Instead, it generates responses solely based on the patterns it has learned from the text it was trained on up until its knowledge cutoff date (OpenAI, 2023). In the past, clinicians have turned to their textbooks or internet searches to research diseases and prove or disprove hypotheses. However, these traditional methods pressure the clinician to direct the search for information and leave potential diseases unexplored if they exist outside the clinician’s sphere of awareness. Figure 1 outlines a repeatable process for integrating AI models in clinical decision-making and diagnostic processes.
Fig. 1
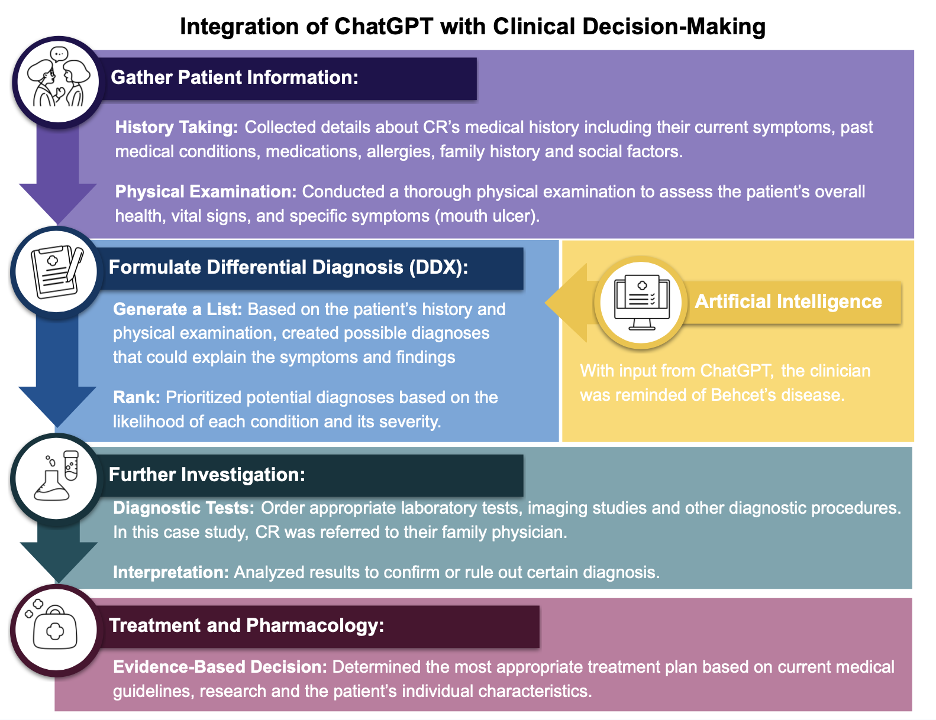
The United States Medical Licensing Exam (USMLE) is a challenging exam that requires hundreds of hours of dedicated study time by medical students. A recent study showed that ChatGPT can perform at or near the passing threshold for the USMLE without specialized training or reinforcement (Kung, 2023). The model is sufficiently trained to meet regulated standards of medical knowledge. The questions on the USMLE are textually and conceptually dense. However, the study showed that not only were ChatGPT’s explanations on the exam acceptable, but they also contained non-obvious insight. ChatGPT’s approach to the USMLE indicates its value as a tool for clinicians. In addition to drawing on their education, professional experience and schema when diagnosing patients, insight from ChatGPT has the potential to inform the clinician of additional considerations better.
Considerations when using AI: Privacy Concerns
However, AI models such as ChatGPT are not perfect. The technology still comes with the risk of misdiagnosis and should never be used in place of a medical professional. LLMs also suffer from their own biases in decision-making and biases that stem from the data sets and information they are trained with. Clinical AI is an expanding subset of AI, and researchers show that it must be guided by the principles of trust and explainability, which serve as a current benchmark for evaluating ChatGPT’s responses regarding diseases and medical diagnoses (Kung, 2023).
The use of LLMs also introduces the risk of data privacy issues. Dental offices and health care providers are a growing target for cyber threat factors due to their large amounts of Personal Identifiable Information (PII) (Fritz, 2022). Threat actors understand that a clinician’s reputation is among their most valuable assets, thus making clinics a desirable target for paying large ransoms in exchange for their patient’s PII information not being released publicly.
While threat actors have traditionally targeted databases of patient information, compromising a clinician’s AI tool credentials and reviewing their past searches is a straightforward means of stealing information and has particularly devastating consequences if the clinician has entered patient PII. To mitigate this risk, clinicians who use AI tools are advised to create a separate account with a strong password for their professional queries. PII should never be input into the AI tool, and data that must be entered to inform AI’s response should be anonymized. In our case study, the only information required about the patient to provide an insightful response was their symptoms–note that neither age nor gender was provided. The details about the patient the clinician has access to should be used to eliminate solutions from the possible diseases generated by AI. For example, the clinician knew that CR was an adult–ChatGPT likely would not have suggested Hand, Foot, and Mouth disease (a viral illness in infants) had the model accessed this information. However, from a cybersecurity standpoint, it was wise of the clinician to provide the least amount of information possible and then quickly eliminate irrelevant results based on their complete understanding of the patient’s background and situation.
Clinicians should also be aware that their inputs to AI tools are retained for 30 days. OpenAI has implemented safety measures to prevent the generation of PII as part of its content filtering mechanisms, but this does not guarantee that personal information will not penetrate other users’ responses based on prompts (OpenAI, 2023). Moreover, while user inputs are no longer used to improve the model’s performance through direct training, clinicians should still be extremely cautious and never share sensitive information.
An essential final step in using AI tools securely as a clinician is educating staff about the potential for data leaks and clearly outlining an implementation plan for the risk mitigation strategies outlined in this discussion. Especially as ChatGPT and generative AI continue to evolve and newer versions of the platform are released, clinicians must ensure that they use the technology to optimize their diagnostic processes without compromising data security.
The Importance of Diagnosis
In this case, AI was able to assist a periodontist in finding a possible diagnosis for CR. While it is not in the periodontist’s training or expertise to manage Behcet’s disease, narrowing in on possible differential diagnosis improved CR’s peace of mind and will direct further investigations. The importance of a medical label may be controversial, but research shows that for many individuals, having a formal diagnosis allows for improved self-efficacy (Novakotch et al., 2015). In a survey conducted in the United States and the United Kingdom, information on diagnosis was rated as one of the most important factors for patients with vasculitis (Mooney et al., 2014). Also, earlier diagnosis allows for earlier intervention, possibly preventing multi-organ involvement, as is often the case in Behcet’s disease.
Conclusion
The expertise of clinicians guides medical diagnosis. An essential factor in this decision-making is the prevalence of any disease. Clinical gestalt–a nebulous concept for new medical learners–improves with experience. However, with time, the familiarity with and subsequent expectation of certain diseases can bias human decision-making. AI can improve healthcare outcomes by bringing rare diseases to a clinician’s awareness, allowing for earlier diagnosis and intervention. In our case study, ChatGPT redirected our search for a horse in the North American Plains to a zebra in the African Savanna.
The periodontist, although experienced, faced difficulty identifying the underlying cause due to the rarity of the disease and the fact that its management primarily lies outside of a periodontist’s scope of practice. However, by obtaining a detailed history from the patient, the periodontist could draw upon a vast repository of medical literature and case studies through ChatGPT to determine the potential diagnosis of Behcet’s disease. With this knowledge, management with a steroid mouthwash was deemed viable, along with the further recommendation to CR’s family physician for additional workup. A brief dialogue with AI allowed the periodontist to bring direction to CR’s medical management and validate CR’s clinical symptoms.
However, integrating AI tools like ChatGPT into medical practice raises critical ethical considerations concerning patient privacy and data security. As medical information is shared with AI models, ensuring patient data confidentiality becomes paramount. Moreover, the potential limitations of AI should be acknowledged. ChatGPT’s knowledge is based on information available up to a specific time, and it might not have access to the most recent medical developments. Additionally, AI models lack the clinical intuition, empathy, and context awareness that human medical practitioners possess, making it imperative that trained professionals interpret AI-generated insights – the integration of the clinician’s skillset and AI’s knowledge allowed for the successful management of CR.
In summary, AI tools hold promise as a supportive tool for healthcare practitioners in diagnosing rare medical conditions. It can expedite information retrieval, assist in generating differential diagnoses, and facilitate interdisciplinary collaboration. Responsible and ethical implementation, encompassing patient privacy safeguards and a thorough understanding of AI’s capabilities and limitations, is essential to harness the full potential of AI in improving patient care while upholding the highest standards of medical practice.
Appendix A: ChatGPT Interaction
An interaction was undertaken with ChatGPT, an AI language model developed by OpenAI. This interaction aimed to gather insights and possible causes based on symptoms the patient was experiencing. The following question was posed to ChatGPT by the clinician:
Question:
What medical conditions are associated with a painful mouth, oral ulcers and a facial skin rash?
ChatGPT Response:
There are several conditions that could be associated with symptoms like a painful mouth, oral ulcers, and a facial skin rash, some of them are:
- Behçet’s disease: This rare disorder leads to inflammation throughout the body. It can result in painful mouth sores, genital sores, inflammation in eyes, and skin problems.
- Herpes simplex virus (HSV): Oral herpes, often referred to as cold sores, can cause painful sores on or around the mouth. Herpes can also occasionally cause skin rashes.
- Lupus: This is a systemic autoimmune disease that occurs when your body’s immune system attacks your own tissues and organs. Inflammation caused by lupus can affect many different body systems, including your skin and mouth. Oral ulcers and a butterfly-shaped rash across the cheeks and nose are common symptoms.
- Pemphigus Vulgaris: This is a rare autoimmune condition that can cause painful blisters and sores in the mouth and on the skin of the face and other parts of the body.
- Stevens-Johnson syndrome/Toxic epidermal necrolysis: These are severe skin reactions, often to medications or infections, that can include symptoms like a painful mouth and oral ulcers, face rash, and widespread skin pain.
- Hand, Foot and Mouth Disease: This is a common viral illness that usually affects infants and children younger than 5 years old. It can cause mouth ulcers and a rash on the hands and feet.
- Erythema Multiforme: This is a skin reaction that can be triggered by an infection or medication. It often involves red, painful lesions on the skin and the mucous membranes, including inside the mouth.
- Lichen Planus: It’s a condition that can cause swelling and irritation in the skin, hair, nails and mucous membranes. On the skin, lichen planus usually appears as purplish, itchy, flat bumps. Inside the mouth, it forms white patches, sores or ulcers.
It’s important to note that these symptoms could be associated with a variety of conditions, and this list is not exhaustive. These symptoms warrant medical evaluation. If you or someone else has these symptoms, it’s advised to seek professional medical help.
Note: The interaction with ChatGPT was conducted on May 17th, 2023, using the GPT-4 architecture.
Oral Health welcomes this original article.
References
- Adil, A., Goyal, A., & Quint, J. M. (2023, February 22). Behcet Disease. In StatPearls [Internet]. Treasure Island (FL): StatPearls Publishing. Available from: https://www.ncbi.nlm.nih.gov/books/NBK470257/
- Calamia, K. T., Wilson, F. C., Icen, M., Crowson, C. S., Gabriel, S. E., & Kremers, H. M. (2009). Epidemiology and clinical characteristics of Behçet’s disease in the US: a population-based study. Arthritis Rheum, 61(5), 600-604.
- Densen, P. (2011). Challenges and opportunities facing medical education. Trans Am Clin Climatol Assoc, 122, 48-58. PMID: 21686208; PMCID: PMC3116346.
- Duarte, F. (2023). Number of CHATGPT users (2023) [Internet]. Exploding Topics. Available from: https://explodingtopics.com/blog/chatgpt-users#
- Fritz, P., & Fritz, C. (2022). Cybersecurity & Data Protection in Dentistry: A global threat [Internet]. Available from: https://www.oralhealthgroup.com/features/cybersecurity-data-protection-in-dentistry-a-global-threat/
- Kung, T. H., Cheatham, M., Medenilla, A., Sillos, C., Leon, L. D., Elepaño, C., … & Yang, H. (2023). Performance of ChatGPT on USMLE: Potential for AI-assisted medical education using large language models. PLoS Digital Health. Available from: https://journals.plos.org/digitalhealth/article?id=10.1371/journal.pdig.0000198
- Melikoglu, M., Kural-Seyahi, E., Tascilar, K., & Yazici, H. (2008). The unique features of vasculitis in Behçet’s syndrome. Clin Rev Allergy Immunol, 35(1-2), 40-46.
- Mooney, J., Spalding, N., Poland, F., Grayson, P., Leduc, R., McAlear, C. A., … & Watts, R. A. (2014). The informational needs of patients with ANCA-associated vasculitis – development of an informational needs questionnaire. Rheumatology, 53(8), 1414-1421.
- Novakovich, E., & Grayson, P. C. (2015). What matters for patients with vasculitis?. La Presse Médicale, 44(6), e267-e272.
- OpenAI. (2023). ChatGPT, response to author query. (Aug 3 version) [Large language model]. Available from: https://chat.openai.com/chat
- Sevilla, J., Heim, L., Ho, A., Besiroglu, T., Hobbhahn, M., & Villalobos, P. (2022). Compute trends across three eras of machine learning. In 2022 International Joint Conference on Neural Networks (IJCNN) (pp. 1-8). IEEE.
- Zouboulis, C. C., & May, T. (2003). Pathogenesis of Adamantiades-Behçet’s disease. Adv Exp Med Biol, 528, 161-171.
About the Authors
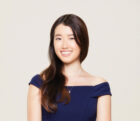
Sophia Pei is a medical student at McMaster University’s Michael DeGroote School of Medicine. She has completed her Master’s and Bachelor of Science degrees in Quantitative and Computational Biology at the University of Southern California.
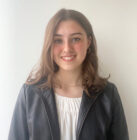
Charlotte Fritz is completing a Bachelor of Science in Computer Engineering at the University of Toronto, with minors in Artificial Intelligence and Business. She is a Cybersecurity Associate at Grant Thornton LLP and a member of Women in Defense and Security.